The relevance of Artificial Intelligence and Machine Learning for tackling carbon emissions
Fri, 07/10/2022 - 18:10
Go Green
In this article, we reflect on Artificial Intelligence (AI) and its cognitive domain, Machine Learning (ML), and how these technologies may be the future for controlling greenhouse gas emission levels in the transport and logistics sector.
In recent years, greenhouse gas (GHG) emissions and their negative impacts on the global climate have been often debated. Fortunately, tangible steps have been taken to reduce these emissions, as shown by the German Climate Action Plan 2050 and the European Green Deal. In addition, global corporate endeavors like the Carbon Disclosure Project (CDP), which helps companies and cities disclose their environmental impact, and other science-based initiatives have also been implemented to reduce companies' carbon emissions.
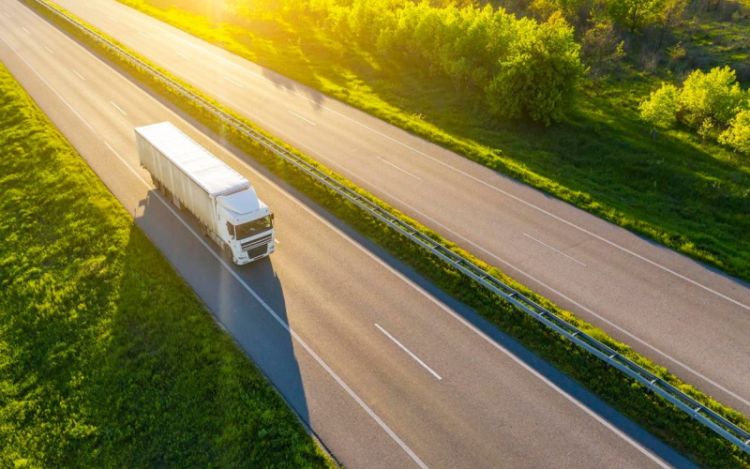
Along with these government and corporate initiatives, Artificial Intelligence (AI) and its cognitive domain, Machine Learning (ML), are also increasingly used to mitigate climate change and are driving the future of GHG emission levels for private individuals and organizations. As for the transport and logistics sector, we believe ML tools can also be particularly helpful in making data-driven decisions regarding carbon emissions.
Over time, using Artificial Intelligence and Machine Learning in the transport and logistics sector has successfully tackled carbon emissions. Here are some examples:
Support in managing transportation activities
Due to demand imbalances, time constraints, or insufficient planning, approximately 30% of transports are (partially) empty runs. In recent years Machine Learning has helped companies reduce their transport activity and emissions by geographically grouping shippers and goods destinations and detecting breaks in transportation routes. This technology has also made it possible to anticipate complex cargo size combinations, transport type, route coverage, and service characteristics.Vehicle analysis and enhanced transport efficiency
Machine Learning algorithms have also been used to perform predictive analyses of vehicles and to accurately assemble their components, resulting in better vehicle design and functionality and more efficient freight transport. In addition, the use of ML for vehicle analysis has had a positive impact on emissions reduction: By accessing a large volume of data on all vehicle components, companies have a superior understanding of their vehicles and achieve more efficient driving with less vehicle wear and fewer emissions.Support for electric vehicles
The most well-known application of ML tools in the transportation and logistics industry is undoubtedly electric vehicles (EVs), in which object and pattern detection is often used to improve safety and driving behavior. ML methods applied to EVs are also beneficial for emission control and climate change mitigation, as they provide valuable support to the recharging process of EVs, allowing them to detect anomalies early and preventing charging failures. Also, with its ability to learn continuously from performance data, ML methods can make the vehicle production process more cost-effective.Cargo security, arrival time, and truck identification at facilities
Regarding logistics issues like cargo security, arrival times, and vehicle monitoring and identification, Machine Learning methods are also often used in logistics facilities. These technologies make it easy to locate a vehicle at a facility and minimize any potential mistakes while planning the pickup and unloading of cargo. Additionally, by using sorting and grouping standards, ML tools provide improved estimates of transport duration and arrival time, reducing costs and improving workflow in shipyards, logistics centers, and other facilities. As a result, emissions at these locations are reduced.Using Artificial Intelligence and Machine Learning in the transport and logistics sector is predicted to be one of the most sustainable strategies to meet future climate goals. Although these technologies are still being used on a small scale in the industry, they represent a real opportunity to reduce GHG emissions. Companies are beginning to use them to increase their data generation ability and make their operations more efficient.
Source:
APPANION
Post tags:
- Frotcom
- Truck emissions
- Fleet emissions
- CO2 emissions
- Carbon emissions
- Greenhouse gas
- Green Fleet
- Go Green
- Climate change
- Climate Action Plan 2050
- European Green Deal
- Machine Learning
- Artificial intelligence
- IoT
- Internet of Things
- Connected vehicles
- Electric vehicles
- Transport efficiency
- Efficient Driving
- Fleet management
- Fleet managers